Research Interests
I'm interested in computer vision and machine learning, especially scene understanding, 3D reconstruction and multimedia analysis. Most of my research are about how to understand the semantic content and infer the physical information from images and videos.
|
News
[2025.2.27] Two papers are accepted by CVPR 2025.
[2024.12.9] Two papers are accepted by AAAI 2025.
[2024.7.1] Two papers are accepted by ECCV 2024.
[2024.5.15] Call for papers on IEEE TMM Special Issue on Large Multi-modal Models for Dynamic Visual Scene Understanding, please refer to https://signalprocessingsociety.org/blog/ieee-tmm-special-issue-large-multi-modal-models-dynamic-visual-scene-understanding.
|
|
Global-Local Tree Search in VLMs for 3D Indoor Scene Generation
Wei Deng,
Mengshi Qi*,
Huadong Ma
CVPR, 2025
pdf /
arxiv /
press /
bibtex
This paper considers 3D indoor scene generation as a planning problem subject to spatial and layout common sense constraints. To solve the problem with a VLM, we propose a new global-local tree search algorithm.
|
|
T2SG: Traffic Topology Scene Graph for Topology Reasoning in Autonomous Driving
Changsheng Lv,
Mengshi Qi*,
Liang Liu,
Huadong Ma
CVPR, 2025
pdf /
arxiv /
press /
bibtex
In this paper, we defined a novel Traffic Topology Scene Graph, a unified scene graph explicitly modeling the lane, controlled and guided by different road signals (e.g., right turn), and topology relationships among them, which is always ignored by previous high-definition (HD) mapping methods.
|
|
VIoTGPT: Learning to Schedule Vision Tools towards Intelligent Video Internet of Things
Yaoyao Zhong,
Mengshi Qi*,
Rui Wang,
Yuhan Qiu,
Yang Zhang,
Huadong Ma
AAAI, 2025   (Oral Presentation)
pdf /
arxiv /
code /
press /
bibtex
In this paper, to address the challenges posed by the fine-grained and interrelated vision tool usage of VIoT, we build VIoTGPT, the framework based on LLMs to correctly interact with humans, query knowledge videos, and invoke vision models to accomplish complicated tasks.
|
|
Efficient Cloud-edge Collaborative Inference for Object Re-identification
Chuanming Wang,
Yuxin Yang,
Mengshi Qi*,
Huanhuan Zhang,
Huadong Ma
AAAI, 2025
pdf /
arxiv /
code /
press /
bibtex
In this paper, we pioneer a cloud-edge collaborative inference framework for ReID systems and particularly propose a distribution-aware correlation modeling network (DaCM) to make the desired image return to the cloud server as soon as possible via learning to model the spatial-temporal correlations among instances.
|
|
Semi-Supervised Teacher-Reference-Student Architecture for Action Quality Assessment
Wulian Yun,
Mengshi Qi*,
Fei Peng,
Huadong Ma
ECCV, 2024
pdf /
press /
video /
code /
arxiv /
bibtex
In this paper, we propose a novel semi-supervised method, which can be utilized for better assessment of the AQA task by exploiting a large amount of unlabeled data and a small portion of labeled data.
|
|
Decomposed Vector-Quantized Variational Autoencoder for Human Grasp Generation
Zhe Zhao,
Mengshi Qi*,
Huadong Ma
ECCV, 2024
pdf /
press /
video /
code /
arxiv /
bibtex
In this paper, we propose a novel Decomposed Vector-Quantized Variational Autoencoder~(DVQ-VAE) to address this limitation by decomposing hand into several distinct parts and encoding them separately.
|
|
SGFormer: Semantic Graph Transformer for Point Cloud-based 3D Scene Graph Generation
Changsheng Lv,
Mengshi Qi*,
Xia Li,
Zhengyuan Yang,
Huadong Ma
AAAI, 2024
pdf /
press /
video /
code /
arxiv /
bibtex
In this paper, we propose the semantic graph Transformer (SGT) for 3D scene graph generation. The task aims to parse a cloud point-based scene into a semantic structural graph, with the core challenge of modeling the complex global structure.
|
|
Weakly-Supervised Temporal Action Localization by Inferring Salient Snippet-Feature
Wulian Yun,
Mengshi Qi*,
Chuanming Wang,
Huadong Ma
AAAI, 2024
pdf /
press /
video /
code /
arxiv /
bibtex
In this paper, we propose a novel weakly supervised temporal action localization method by inferring salient snippet-feature.
|
|
RDFC-GAN: RGB-Depth Fusion CycleGAN for Indoor Depth Completion
Haowen Wang,
Zhengping Che,
Mingyuan Wang,
Zhiyuan Xu,
Xiuquan Qiao,
Mengshi Qi*,
Feifei Feng,
Jian Tang
IEEE Transactions on Pattern Analysis and Machine Intelligence (TPAMI), 2024   (An extension of our CVPR 22' paper)
pdf /
arxiv /
press /
code /
bibtex
In this paper, we design a novel two-branch end-to-end fusion network named RDFC-GAN, which takes a pair of RGB and incomplete depth images as input to predict a dense and completed depth map.
|
|
Mutual Distillation Learning For Person Re-Identification
Huiyuan Fu,
Kuilong Cui,
Chuanming Wang,
Mengshi Qi*,
Huadong Ma
IEEE Transactions on Multimedia (TMM), 2024
pdf /
arxiv /
press /
code /
bibtex
In this paper, we propose a novel approach, Mutual Distillation Learning For Person Re-identification (termed as MDPR), which addresses the challenging problem from multiple perspectives within a single unified model, leveraging the power of mutual distillation to enhance the feature representations collectively.
|
|
Disentangled Counterfactual Learning for Physical Audiovisual Commonsense Reasoning
Changsheng Lv,
Shuai Zhang,
Yapeng Tian,
Mengshi Qi*,
Huadong Ma
NeurIPS, 2023
pdf /
press /
video /
arxiv /
code /
bibtex
In this paper, we propose a Disentangled Counterfactual Learning~(DCL) approach for physical audiovisual commonsense reasoning. The task aims to infer objects' physics commonsense based on both video and audio input, with the main challenge is how to imitate the reasoning ability of humans.
|
|
Unsupervised Self-Driving Attention Prediction via Uncertainty Mining and Knowledge Embedding
Pengfei Zhu,
Mengshi Qi*,
Xia Li,
Weijian Li,
Huadong Ma
ICCV, 2023
pdf /
press /
video /
arxiv /
code /
bibtex
In this paper, we are the first to introduce an unsupervised way to predict self-driving attention by uncertainty modeling and driving knowledge integration.
|
|
GaitReload: A Reloading Framework for Defending Against On-Manifold Adversarial Gait Sequences
Peilun Du,
Xiaolong Zheng,
Mengshi Qi,
Huadong Ma
IEEE Transactions on Information Forensics and Security (TIFS), 2023
pdf /
press /
bibtex
In this paper, we propose GaitReload, a post-processing adversarial defense method to defend against AWP for the gait recognition model with sequenced inputs.
|
|
RGB-Depth Fusion GAN for Indoor Depth Completion
Haowen Wang,
Mingyuan Wang,
Zhengping Che,
Zhiyuan Xu,
Xiuquan Qiao,
Mengshi Qi,
Feifei Feng,
Jian Tang
CVPR , 2022
pdf /
press /
bibtex
In this paper, we design a novel two-branch end-to-end fusion network, which takes a pair of RGB and incomplete depth images as input to predict a dense and completed depth map.
|
|
Semantics-Aware Spatial-Temporal Binaries for Cross-Modal Video Retrieval
Mengshi Qi,
Jie Qin,
Yi Yang,
Yunhong Wang,
Jiebo Luo
IEEE Transactions on Image Processing (TIP), 2021
pdf /
press /
bibtex
In this paper, we propose a novel binary representation learning framework, named Semantics-aware Spatial-temporal Binaries (S2Bin), which simultaneously considers spatial-temporal context and semantic relationships for cross-modal video retrieval. By exploiting the semantic relationships between two modalities, S2Bin can efficiently and effectively generate binary codes for both videos and texts. In addition, we adopt an iterative optimization scheme to learn deep encoding functions with attribute-guided stochastic training.
|
|
Latent Memory-augmented Graph Transformer for Visual Storytelling
Mengshi Qi,
Jie Qin,
Di Huang,
Zhiqiang Shen,
Yi Yang,
Jiebo Luo
ACM MM, 2021   (Oral Presentation)
pdf /
press /
video /
bibtex
In this paper, we present a novel Latent Memory-augmented Graph Transformer (LMGT), a Transformer based framework including a designed graph encoding module and a latent memory unit for visual story generation.
|
|
Imitative Non-Autoregressive Modeling for Trajectory Forecasting and Imputation
Mengshi Qi,
Jie Qin,
Yu Wu,
Yi Yang
CVPR, 2020
pdf /
press /
video /
bibtex
In this paper, we proposed a novel Imitative Non-Autoregressive Modeling method to bridge the performance gap between autoregressive and non-autoregressive models for temporal sequence forecasting and imputation. Our proposed framework leveraged an imitation learning fashion including two parts, i.e., a recurrent conditional variational autoencoder (RC-VAE) demonstrator and a nonautoregressive transformation model (NART) learner.
|
|
Few-Shot Ensemble Learning for Video Classificaion with SlowFast Memory Networks
Mengshi Qi,
Jie Qin,
Xiantong Zhen,
Di Huang,
Yi Yang,
Jiebo Luo
ACM MM, 2020
pdf /
press /
video /
bibtex
In this paper, we address few-shot video classification by learning an ensemble of SlowFast networks augmented with memory units. Specifically, we introduce a family of few-shot learners based on SlowFast networks which are used to extract informative features at multiple rates, and we incorporate a memory unit into each network to enable encoding and retrieving crucial information instantly.
|
|
STC-GAN: Spatio-Temporally Coupled Generative Adversarial Networks for Predictive Scene Parsing
Mengshi Qi,
Yunhong Wang,
Annan Li,
Jiebo Luo
IEEE Transactions on Image Processing (TIP), 2020
pdf /
press /
bibtex
In this paper, we present a novel Generative Adversarial Networks-based model (i.e., STC-GAN) for predictive scene parsing. STC-GAN captures both spatial and temporal representations from the observed frames of a video through CNN and convolutional LSTM network. Moreover, a coupled architecture is employed to guide the adversarial training via a weight-sharing mechanism and a feature adaptation transform between the future frame generation model and the predictive scene parsing model.
|
|
Sports Video Captioning via Attentive Motion Representation and Group Relationship Modeling
Mengshi Qi,
Yunhong Wang,
Annan Li,
Jiebo Luo
IEEE Transactions on Circuits and Systems fo Video Technology (TCSVT), 2019   (An extension of our MMSports@MM 18' paper)
pdf /
press /
bibtex
In this study, we present a novel hierarchical recurrent neural network based framework with an attention mechanism for sports video captioning, in which a motion representation module is proposed to capture individual pose attribute and dynamical trajectory cluster information with extra professional sports knowledge, and a group relationship module is employed to design a scene graph for modeling players’ interaction by a gated graph convolutional network.
|
|
stagNet: An Attentive Semantic RNN for Group Activity and Individual Action Recognition
Mengshi Qi,
Yunhong Wang,
Jie Qin,
Annan Li,
Jiebo Luo,
Luc Van Gool
IEEE Transactions on Circuits and Systems for Video Technology (TCSVT), 2019   (An extension of our ECCV 18' paper)
pdf /
press /
bibtex
In the paper, we present a novel attentive semantic recurrent neural network (RNN), namely stagNet, for understanding group activities and individual actions in videos, by combining the spatio-temporal attention mechanism and semantic graph modeling. Specifically, a structured semantic graph is explicitly modeled to express the spatial contextual content of the whole scene, which is afterward further incorporated with the temporal factor through structural- RNN.
|
|
Attentive Relational Networks for Mapping Images to Scene Graphs
Mengshi Qi*,
Weijian Li*,
Zhengyuan Yang,
Yunhong Wang,
Jiebo Luo
CVPR, 2019
pdf /
arxiv /
press /
bibtex
In this study, we propose a novel Attentive Relational Network that consists of two key modules with an object detection backbone to approach this problem. The first module is a semantic transformation module utilized to capture semantic embedded relation features, by translating visual features and linguistic features into a common semantic space. The other module is a graph self-attention module introduced to embed a joint graph representation through assigning various importance weights to neighboring nodes.
|
|
KE-GAN: Knowledge Embedded Generative Adversarial Networks for Semi-Supervised Scene Parsing
Mengshi Qi,
Yunhong Wang,
Jie Qin,
Annan Li
CVPR, 2019
pdf /
press /
bibtex
In this paper, we propose a novel Knowledge Embedded Generative Adversarial Networks, dubbed as KE-GAN, to tackle the challenging problem in a semi-supervised fashion. KE-GAN captures semantic consistencies of different categories by devising a Knowledge Graph from the large-scale text corpus.
|
|
stagNet: An Attentive Semantic RNN for Group Activity Recognition
Mengshi Qi,
Jie Qin,
Annan Li,
Yunhong Wang,
Jiebo Luo,
Luc Van Gool
ECCV, 2018
pdf /
press /
bibtex
We propose a novel attentive semantic recurrent neural network (RNN), dubbed as stagNet, for understanding group activities in videos, based on the spatio-temporal attention and semantic graph.
|
|
Sports Video Captioning by Attentive Motion Representation based Hierarchical Recurrent Neural Networks
Mengshi Qi,
Yunhong Wang,
Annan Li,
Jiebo Luo
MMSports@MM, 2018   (Oral Presentation)
pdf /
press /
video /
bibtex
In this paper, we present a novel hierarchical recurrent neural network (RNN) based framework with an attention mechanism for sports video captioning. A motion representation module is proposed to extract individual pose attribute and group-level trajectory cluster information.
|
|
Online Cross-modal Scene Retrieval by Binary Representation and Semantic Graph
Mengshi Qi,
Yunhong Wang,
Annan Li
ACM MM, 2017
pdf /
press /
bibtex
We propose a new framework for online cross-modal scene retrieval based on binary representations and semantic graph. Specially, we adopt the cross-modal hashing based on the quantization loss of different modalities. By introducing the semantic graph, we are able to extract wealthy semantics and measure their correlation across different modalities.
|
|
DEEP-CSSR: Scene Classification using Category-specific Salient Region with Deep Features
Mengshi Qi,
Yunhong Wang
IEEE ICIP, 2016   (Oral Presentation)
pdf /
press /
bibtex
In this paper, we introduce a novel framework towards scene classification using category-specific salient region(CSSR) with deep CNN features, called Deep-CSSR.
|
|
Human Grasp Generation for Rigid and Deformable Objects with Decomposed VQ-VAE
Mengshi Qi*,
Zhe Zhao,
Huadong Ma
Arxiv, 2025   (An extension of our ECCV 24' paper)
pdf /
arxiv /
code /
press /
bibtex
In this paper, we propose a novel improved Decomposed Vector-Quantized Variational Autoencoder (DVQ-VAE-2) with a new Mesh UFormer as the backbone network to extract the hierarchical structural representations from the mesh and propose a new normal vector-guided position encoding to simulate the hand-object deformation.
|
|
Robust Disentangled Counterfactual Learning for Physical Audiovisual Commonsense Reasoning
Mengshi Qi*,
Changsheng Lv,
Huadong Ma
Arxiv, 2025   (An extension of our NeurIPS 23' paper)
pdf /
arxiv /
code /
data /
press /
bibtex
In this paper, we propose a new Robust Disentangled Counterfactual Learning (RDCL) approach for physical audiovisual commonsense reasoning.
|
|
Towards Robust Unsupervised Attention Prediction in Autonomous Driving
Mengshi Qi*,
Xiaoyang Bi,
Pengfei Zhu,
Huadong Ma
Arxiv, 2025   (An extension of our ICCV 23' paper)
pdf /
arxiv /
code /
data /
press /
bibtex
In this paper, we propose a novel robust unsupervised attention prediction method, which includes an Uncertainty Mining Branch, Knowledge Embedding Block, and RoboMixup, a novel data augmentation method to improve robustness against corruption and central bias.
|
|
Action Quality Assessment via Hierarchical Pose-guided Multi-stage Contrastive Regression
Mengshi Qi*,
Hao Ye,
Jiaxuan Peng,
Huadong Ma
Arxiv, 2025   (An extension of our ICASSP 24' paper)
pdf /
arxiv /
code /
press /
bibtex
In this paper, we propose a novel action quality assessment method through hierarchically pose-guided multi-stage contrastive regression.
|
|
VLM-Assisted Continual learning for Visual Question Answering in Self-Driving
Yuxin Lin,
Mengshi Qi*,
Liang Liu,
Huadong Ma
Arxiv, 2025
pdf /
arxiv /
code /
press /
bibtex
In this paper, we present a novel continual learning framework that combines VLMs with selective memory replay and knowledge distillation, reinforced by task-specific projection layer regularization.
|
|
Target-driven Self-Distillation for Partial Observed Trajectories Forecasting
Pengfei Zhu,
Peng Shu,
Mengshi Qi*,
Liang Liu,
Huadong Ma
Arxiv, 2025
pdf /
arxiv /
code /
press /
bibtex
In this paper, we introduce a Target-driven Self-Distillation method (TSD) for motion forecasting. Our method leverages predicted accurate targets to guide the model in making predictions under partial observation condition.
|
|
Towards Balanced Continual Multi-Modal Learning in Human Pose Estimation
Jiaxuan Peng,
Mengshi Qi*,
Dong Zhao,
Huadong Ma
Arxiv, 2025
pdf /
arxiv /
code /
press /
bibtex
In this paper, we introduce a novel balanced continual multi-modal learning method for 3D HPE, which harnesses the power of RGB, LiDAR, mmWave, and WiFi.
|
|
A New Teacher-Reviewer-Student Framework for Semi-supervised 2D Human Pose Estimation
Wulian Yun,
Mengshi Qi*,
Fei Peng,
Huadong Ma
Arxiv, 2025
pdf /
arxiv /
code /
press /
bibtex
In this paper, we propose a novel semi-supervised 2D human pose estimation method by utilizing a newly designed Teacher-Reviewer-Student framework.
|
|
Learning Group Interactions and Semantic Intentions for Multi-Object Trajectory Prediction
Mengshi Qi*,
Yuxin Yang,
Huadong Ma
Arxiv, 2024
pdf /
arxiv /
code /
press /
bibtex
In this paper, we propose a novel diffusion-based trajectory prediction framework that integrates group-level interactions into a conditional diffusion model, enabling the generation of diverse trajectories aligned with specific group activity.
|
|
Improving Batch Normalization with TTA for Robust Object Detection in Self-Driving
Dacheng Liao,
Mengshi Qi*,
Liang Liu,
Huadong Ma
Arxiv, 2024
pdf /
arxiv /
press /
bibtex
In this paper, we introduce a LearnableBN layer based on Generalized-search Entropy Minimization (GSEM) method, and a new semantic-consistency based dual-stage-adaptation strategy.
|
|
Adversarial Contrastive Learning Based Physics-Informed Temporal Networks for Cuffless Blood Pressure Estimation
Rui Wang,
Mengshi Qi*,
Yingxia Shao,
Anfu Zhou,
Huadong Ma
Arxiv, 2024
pdf /
arxiv /
code /
press /
bibtex
In this paper, we introduce a novel physics-informed temporal network~(PITN) with adversarial contrastive learning to enable precise Blood Pressure estimation with very limited data.
|
|
Uncovering the human motion pattern: Pattern Memory-based Diffusion Model for Trajectory Prediction
Yuxin Yang,
Pengfei Zhu,
Mengshi Qi*,
Huadong Ma
Arxiv, 2024
pdf /
arxiv /
press /
bibtex
In this paper, we introduce a novel memory-based method, named Motion Pattern Priors Memory Network. Our method involves constructing a memory bank derived from clustered prior knowledge of motion patterns observed in the training set trajectories.
|
|
Coarse-to-Fine Video Denoising with Dual-Stage Spatial-Channel Transformer
Wulian Yun,
Mengshi Qi*,
Chuanming Wang,
Huiyuan Fu,
Huadong Ma
Arxiv, 2022
pdf /
press /
bibtex
In this paper, we propose a Dual-stage Spatial-Channel Transformer for coarse-to-fine video denoising, which inherits the advantages of both Transformer and CNNs.
|
|
Unsupervised Domain Adaptation with Temporal-Consistent Self-Training for 3D Hand-Object Joint Reconstruction
Mengshi Qi,
Edoardo Remelli,
Mathieu Salzmann,
Pascal Fua
Arxiv, 2020
pdf /
press /
bibtex
In this paper, we introduce an effective approach to exploit 3D geometric constraints within a cycle generative adversarial network (CycleGAN) to perform domain adaptation. Furthermore, we propose to enforce short- and long-term temporal consistency to fine-tune the domain-adapted model in a self-supervised fashion.
|
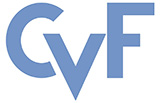 |
Conference Reviewer, ICCV 2019-2023, CVPR 2020-2025, ECCV 2020-2022, ICML 2021-2025, ICLR 2021-2025, NeurIPS 2020-2023, ACM MM 2021-2023
Journal Reviewer, TPAMI, IJCV, TIP, TMM, TCSVT, PR, ACM Computing Surveys
Guest Editor, IEEE TMM Special Issue on Large Multi-modal Models for Dynamic Visual Scene Understanding
Senior PC Member, IJCAI 2021/2023-2025, AAAI 2023-2025
PC Member, AAAI 2020-2022, IJCAI 2020/2022
Area Chair, ICME 2024/2025
IEEE Member, ACM Member, CCF Member, CAAI Member and CSIG Member
|
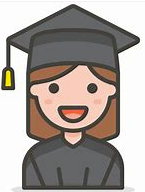 |
Wulian Yun (PhD student, 2020-), co-supervised with Prof. Huadong Ma (1 CCF-A paper, 1 CCF-B paper, 2 patents).
Changsheng Lv (PhD student, 2022-) (3 CCF-A papers).
Rui Wang (PhD student, 2023-) (1 CCF-A paper).
Yonghao Zhou (PhD student, 2023-), co-supervised with Prof. Huadong Ma.
Wei Deng (PhD student, 2024-) (1 CCF-A paper).
Dacheng Liao (PhD student, 2024-), co-supervised with Prof. Liang Liu.
Pengfei Zhu (Master student, 2022-) (1 CCF-A paper, 1 CCF-C paper).
Shuai Zhang (Master student, 2022-) (1 CCF-A paper, 1 patent).
Yanshu He (Master student, 2022-), co-supervised with Prof. Huadong Ma.
Yuang Liu (Master student, 2022-), co-supervised with Prof. Liang Liu (1 CCF-C paper, 1 patent).
Yuxin Yang (Master student, 2023-) (1 CCF-A paper and 1 CCF-C paper).
Zhe Zhao (Master student, 2023-) (1 CCF-B paper, 1 patent).
Jiaxuan Peng (Master student, 2023-) (1 patent).
Yuxin Lin (Master student, 2023-), co-supervised with Prof. Liang Liu.
Xiaoyang Bi (Master student, 2024-).
Hao Ye (Master student, 2024-).
Zijian Fu (Master student, 2024-).
Hongwei Ji (Master student, 2024-).
Yeteng Wu (Master student, 2024-).
Bo Gao (Master student, 2024-), co-supervised with Prof. Huanhuan Zhang.
Peng Shu (Master student, 2024-), co-supervised with Prof. Liang Liu.
Zhining Zhang (Master student, 2024-), co-supervised with Prof. Liang Liu.
Shuaikun Liu (Master student, 2025-).
Zhaohong Liu (Master student, 2025-).
Shuo Wang (Master student, 2025-).
|
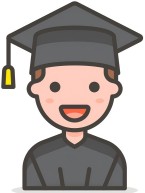 |
Qi An (Master student, 2021-2024), co-supervised with Prof. Huadong Ma (1 CCF-B paper, 1 CCF-A workshop paper), working at POSTAL SAVINGS BANK OF CHINA.
Rongshuai Liu (Master student, 2021-2024), co-supervised with Prof. Huadong Ma (1 CCF-A workshop paper, 1 patent), working at ByteDance.
Kuilong Cui (Master student, 2021-2024), co-supervised with Prof. Huiyuan Fu (1 CCF-B Transactions paper), working at Alibaba.
|
|